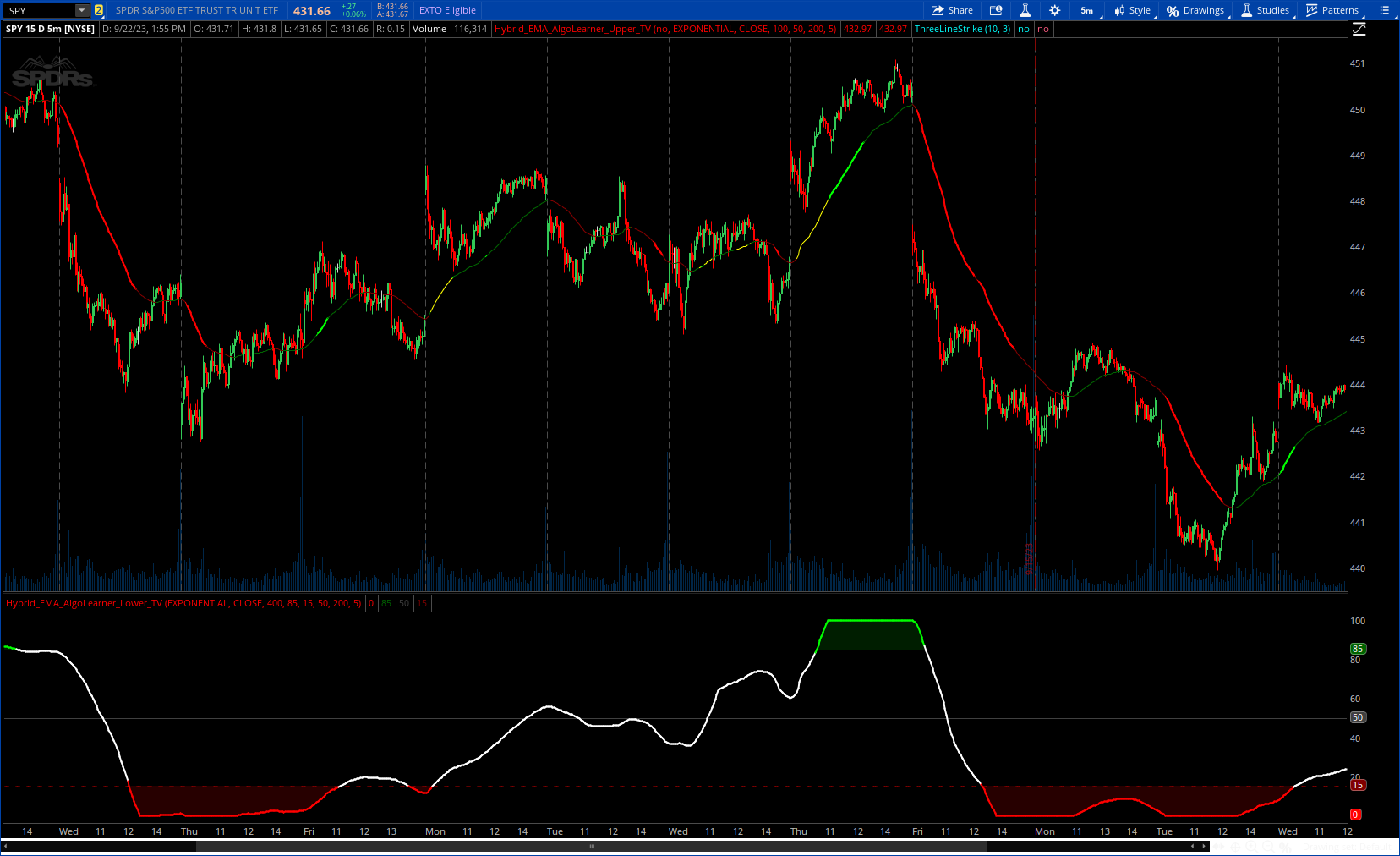
Author Message:
Innovative trading indicator that utilizes a k-NN-inspired algorithmic approach alongside traditional Exponential Moving Averages (EMAs) for more nuanced analysis. While the algorithm doesn't actually employ machine learning techniques, it mimics the logic of the k-Nearest Neighbors (k-NN) methodology. The script takes into account the closest 'k' distances between a short-term and long-term EMA to create a weighted short-term EMA. This combination of rule-based logic and EMA technicals offers traders a more sophisticated tool for market analysis.
More Details : https://www.tradingview.com/v/4jhuhtMN/
- Not Typical -
Upper CODE:
CSS:
#/ https://www.tradingview.com/v/4jhuhtMN/
#// © Uldisbebris
#indicator("Hybrid EMA AlgoLearner", shorttitle="Hybrid EMA AlgoLearner", overlay=false)
# Converted and mod by Sam4Cok@Samer800 - 09/2023
input showSignals = no;
input MovAvgType = AverageType.EXPONENTIAL;
input source = close;
input lookback = 100;
input shortTermPeriod = 50;
input longTermPeriod = 200;
input NumberOfNeighbors = 5;
def na = Double.NaN;
def last = isNaN(Close);
def k = NumberOfNeighbors;
#// Calculate EMAs
def shortEma = MovingAverage(MovAvgType, source, shortTermPeriod);
def longEma = MovingAverage(MovAvgType, source, longTermPeriod);
def shortTermEma = shortEma;
def longTermEma = longEma;
#// Custom k-NN Algorithm for weighted EMA
def dist = fold i = 1 to lookback with p do #// Loop through past 100 data points
max(p, AbsValue(shortTermEma - longTermEma[i]));
def distances = dist;
#def k_distances = fold i1 = 0 to k with p1 do
# max(p1, distances[i1]);
#// Calculate weighted EMA based on closest k distances
def weightEma = fold j = 0 to k with q do
q + shortTermEma[j] * GetValue(distances, j);
def totalWeight = fold j1 = 0 to k with q1 do
q1 + GetValue(distances, j1);
def weightShortTermEma = weightEma / totalWeight;
def WeightedEma = weightShortTermEma;
#/ Instead of all the history, only look at the last N bars.
def cond = close - WeightedEma;# then k+1 else k;
def Predect = fold n = 0 to k with s do
s + if GetValue(cond, n) < GetValue(distances,fold n1 = 0 to n + 1 with s1 do
if GetValue(distances, n1) > GetValue(distances, n1+1) then s1 else s1 +1)
then GetValue(cond, n) else s;
def sumv = Predect;#sum(Predect, k) * 5 ;
def dir = if sumv < 0 then -1 else
if sumv > 0 then 1 else 0;
def bar = barNumber();
def sumDir = fold m = 0 to k+1 with r do
r + dir[m];
def extUp = dir > 0 and sumv > (k + 1);
def extDn = dir < 0 and sumv < (-k - 1);
def secLine = extUp or extDn;
#//== plot
plot emaplot = WeightedEma; # 'Scaled Weighted Short-Term EMA'
emaplot.AssignValueColor(if extUp then Color.GREEN else
if extDn then Color.RED else
if dir > 0 then Color.DARK_GREEN else
if dir < 0 then Color.DARK_RED else Color.YELLOW);
plot emaplot2 = if secLine then WeightedEma else na;
emaplot2.SetLineWeight(2);
emaplot2.AssignValueColor(if extUp then Color.GREEN else Color.RED);
AddChartBubble(showSignals and extUp and !extUp[1] and sumDir > k/2, low, "B", Color.GREEN, no);
AddChartBubble(showSignals and extDn and !extDn[1] and sumDir < k/2, high, "S", Color.RED, yes);
#-- END of CODE
Lower CODE:
CSS:
#/ https://www.tradingview.com/v/4jhuhtMN/
#// © Uldisbebris
#indicator("Hybrid EMA AlgoLearner", shorttitle="Hybrid EMA AlgoLearner", overlay=false)
# Converted and mod by Sam4Cok@Samer800 - 09/2023
declare lower;
input MovAvgType = AverageType.EXPONENTIAL;
input source = close;
input lookbackPeriod = 400;#, 'lookbackPeriod')
input Overbought = 85;
input Oversold = 15;
input shortTermPeriod = 50;
input longTermPeriod = 200;
#// k-N parameter
input NumberOfNeighbors = 5;#, 'K - Number of neighbors')
def na = Double.NaN;
def last = isNaN(Close);
#// Calculate EMAs
def shortTermEma = MovingAverage(MovAvgType, source, shortTermPeriod);
def longTermEma = MovingAverage(MovAvgType, source, longTermPeriod);
#// Custom k-NN Algorithm for weighted EMA
def dist = fold i = 1 to 100 with p do #// Loop through past 100 data points
Max(p, AbsValue(shortTermEma - longTermEma[i]));
def distance = dist;
#// Calculate weighted EMA based on closest k distances
def weightEma = fold j = 0 to NumberOfNeighbors with q do
q + shortTermEma[j] * distance[j];
def totalWeight = fold j1 = 0 to NumberOfNeighbors with q1 do
q1 + distance[j1];
def weightShortTermEma = weightEma / totalWeight;
#/ Instead of all the history, only look at the last N bars.
def minEma = lowest(weightShortTermEma, lookbackPeriod);
def maxEma = highest(weightShortTermEma, lookbackPeriod);
def scaledWeightShortTermEma = (weightShortTermEma - minEma) / (maxEma - minEma) * 100;
def col = if scaledWeightShortTermEma >= Overbought then 1 else
if scaledWeightShortTermEma <= Oversold then -1 else 0;
#//== plot
plot emaplot = scaledWeightShortTermEma; # 'Scaled Weighted Short-Term EMA'
plot upLvl = if last then na else Overbought;
plot midLinePlot = if last then na else 50;
plot dnLvl = if last then na else Oversold;
emaplot.SetLineWeight(2);
emaplot.AssignValueColor(if col > 0 then Color.GREEN else
if col < 0 then Color.RED else Color.WHITE);
upLvl.SetDefaultColor(Color.DARK_GREEN);
midLinePlot.SetDefaultColor(Color.DARK_GRAY);
dnLvl.SetDefaultColor(Color.DARK_RED);
upLvl.SetStyle(Curve.SHORT_DASH);
dnLvl.SetStyle(Curve.SHORT_DASH);
AddCloud(if col>0 then emaplot else na, Overbought, Color.DARK_GREEN);
AddCloud(if col<0 then Oversold else na, emaplot, Color.DARK_RED);
#-- END of CODE